U-M study shows accurate AI models improved diagnostic decisions, but biased models led to serious declines
12:40 PM
Authors |
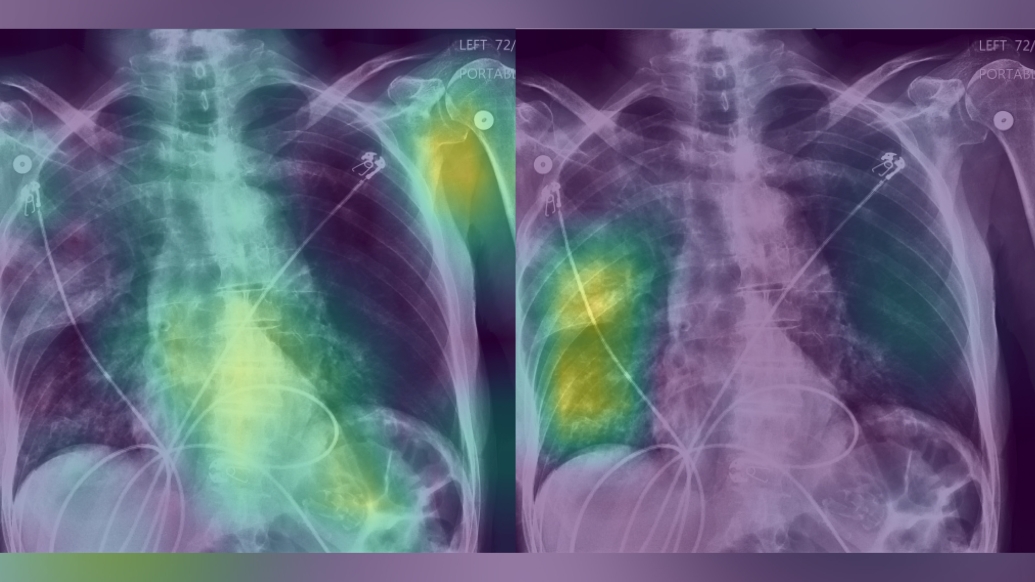
Artificial intelligence (AI) models in healthcare are a double-edged sword, with models improving diagnostic decisions for some demographics but worsening decisions for others when the model has absorbed biased medical data.
Given the very real life and death risks of clinical decision making, researchers and policymakers are taking steps to ensure AI models are safe, secure, and trustworthy—and that their use would lead to improved outcomes.
The U.S. Food and Drug Administration has oversight of software powered by AI, and machine learning used in healthcare and has issued guidance for developers, including a call to make the logic used by AI models to be transparent or explainable so that clinicians can review the underlying reasoning.
However, a new study in JAMA finds that even when provided with AI explanations, clinicians can be fooled by biased AI models.
“The problem is that the clinician has to understand what the explanation is communicating and the explanation itself,” said first author Sarah Jabbour, a Ph.D. candidate in computer science and engineering in the College of Engineering.
The University of Michigan team studied AI models and AI explanations in patients with acute respiratory failure.
“Determining why a patient has respiratory failure can be difficult. In our study, we found clinicians baseline diagnostic accuracy to be around 73 percent,” said Michael Sjoding, M.D., associate professor of internal medicine at the University of Michigan Medical School, a co-senior author on the study.
“During the normal diagnostic process, we think about a patient’s history, lab tests and imaging results, and try to synthesize this information and come up with a diagnosis. It makes sense that a model could help improve accuracy.”
Jabbour, Sjoding, co-senior author Jenna Wiens, Ph.D., associate professor of computer science and engineering and their multidisciplinary team designed a study to evaluate the diagnostic accuracy of 457 hospitalist physicians, nurse practitioners, and physician assistants with and without assistance from an AI model.
Each clinician was asked to make treatment recommendations based on their diagnosis. Half were randomized to receive an AI explanation with the AI model decision, while the other half received only the AI decision with no explanation.
Clinicians were given real clinical vignettes of patients with respiratory failure as well as a rating from the AI model on whether the patient had pneumonia, heart failure, or chronic obstructive pulmonary disease (COPD). In the half randomized to see explanations, the clinician was provided a heatmap, or visual representation, of where the AI model was looking in the chest radiograph, which served as the basis for the diagnosis.
The team found that clinicians who were presented with an AI model trained to make reasonably accurate predictions, but without explanations, had their own accuracy increase by 2.9 percentage points. When provided an explanation, their accuracy increased by 4.4 percentage points.
However, to test whether an explanation could enable clinicians to recognize when an AI model is clearly biased or incorrect, the team also presented clinicians with models intentionally trained to be biased— for example, a model predicting a high likelihood of pneumonia if the patient was 80 years or older
“AI models are susceptible to shortcuts, or spurious correlations in the training data. Given a dataset in which women are underdiagnosed with heart failure, the model could pick up on an association between being female and being at lower risk for heart failure,” explained Wiens.
“If clinicians then rely on such a model, it could amplify existing bias. If explanations could help clinicians identify incorrect model reasoning this could help mitigate the risks.”
When clinicians were shown the biased AI model, however, it decreased their accuracy by 11.3 percentage points and explanations which explicitly highlighted that the AI was looking at non-relevant information (such as low bone density in patients over 80) did not help them recover from this serious decline in performance.
The observed decline in performance aligns with previous studies that find users may be deceived by models, noted the team.
“There’s still a lot to be done to develop better explanation tools so that we can better communicate to clinicians why a model is making specific decisions in a way that they can understand. It’s going to take a lot of discussion with experts across disciplines,” Jabbour said.
The team hopes this study will spur more research into the safe implementation of AI-based models in healthcare across all populations and for medical education surrounding AI and bias.
David Fouhey, Ph.D.; Stephanie Shepard, Ph.D.; Thomas S. Valley, M.D.; Ella A. Kazerooni, M.D., MS; and Nikola Banovic, Ph.D.
This work was supported by grant R01 HL158626 from the National Heart, Lung, and Blood Institute (NHLBI).
Paper cited: “Measuring the Impact of AI in the Diagnosis of Hospitalized Patients: A Randomized Survey Vignette Multicenter Study.” JAMA, DOI:10.1001/jama.2023.22295
Sign up for Health Lab newsletters today. Get medical tips from top experts and learn about new scientific discoveries every week by subscribing to Health Lab’s two newsletters, Health & Wellness and Research & Innovation.
Sign up for the Health Lab Podcast: Add us on Spotify, Apple Podcasts or wherever you get you listen to your favorite shows.
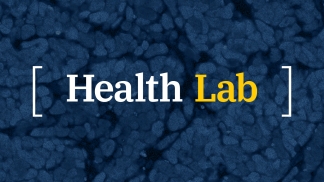
Explore a variety of healthcare news & stories by visiting the Health Lab home page for more articles.

Department of Communication at Michigan Medicine
Want top health & research news weekly? Sign up for Health Lab’s newsletters today!
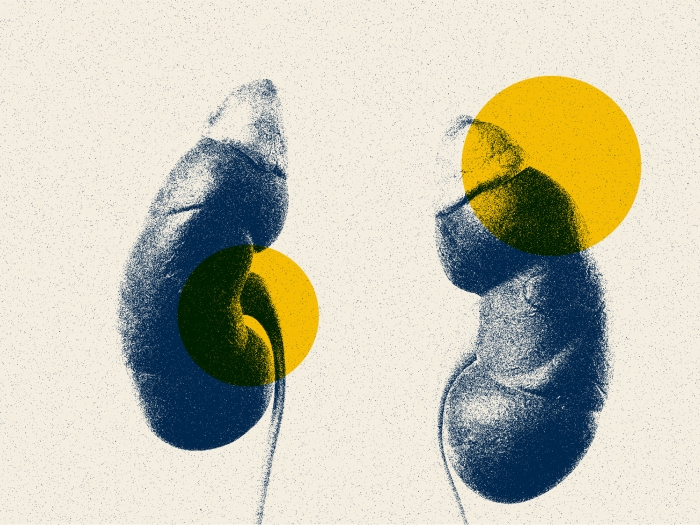
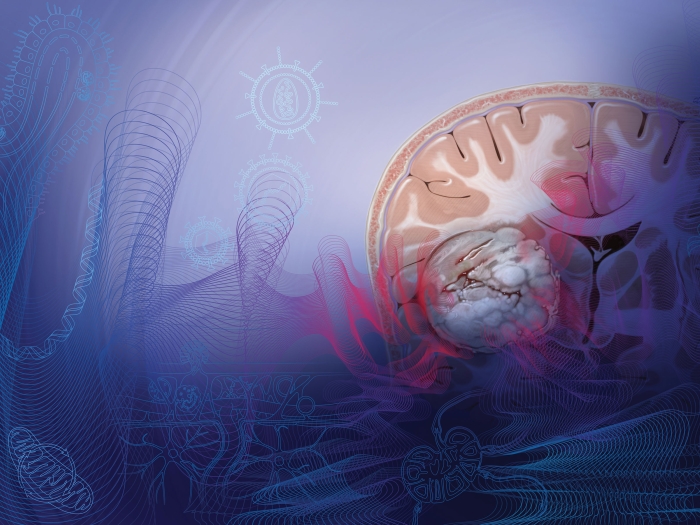
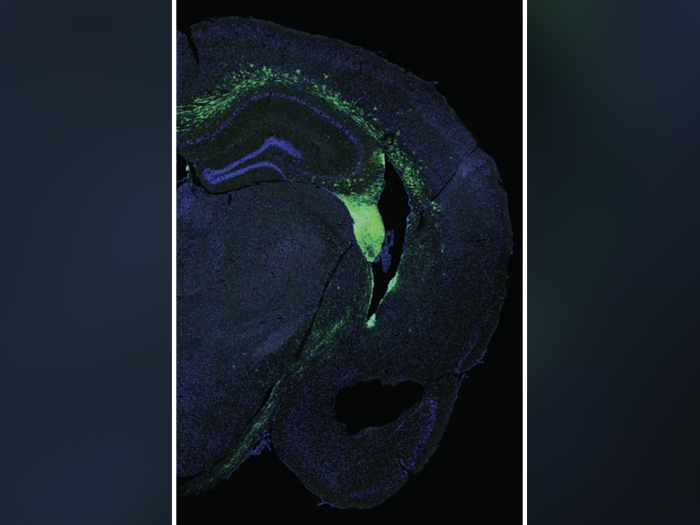
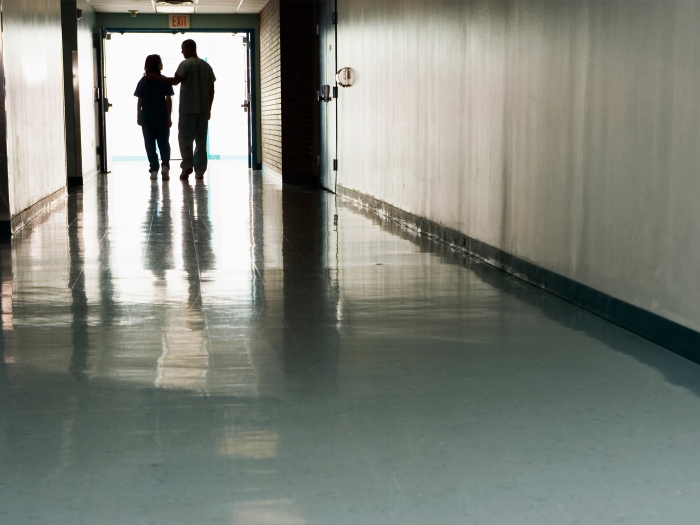
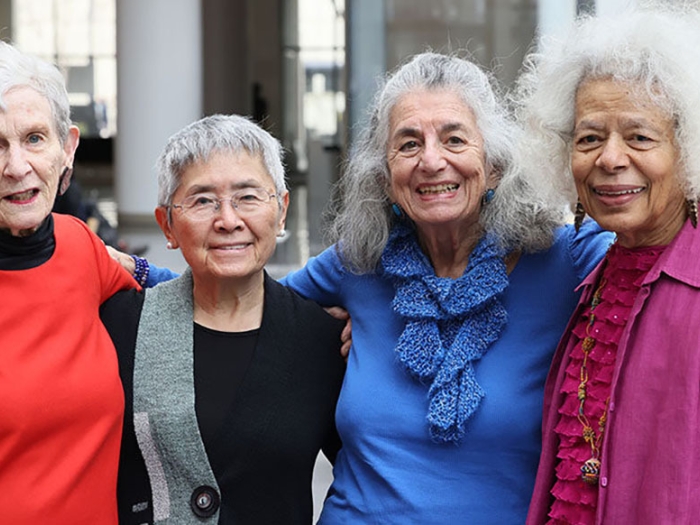
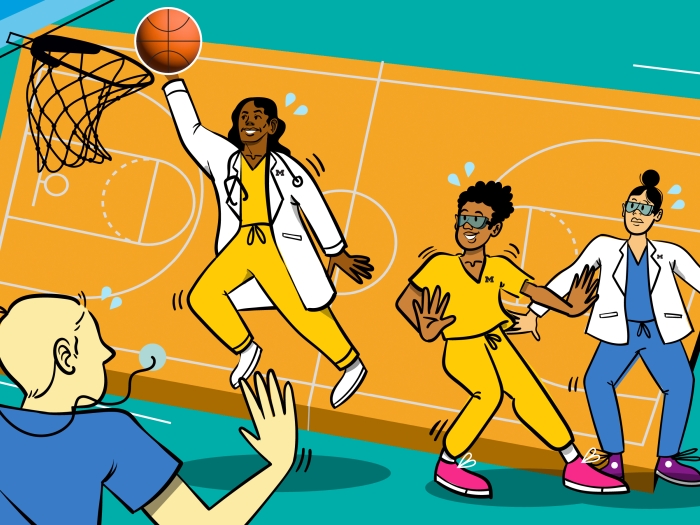