Interview with Lindsay Kobayashi, Ph.D., M.Sc.
5:00 AM
According to an estimate published in 2015, the global prevalence of dementia was projected to nearly triple between 2015 and 2050, growing from 46 million to over 130 million people globally. And of that worldwide share, 70% of those with dementia will be in low- and middle-income countries. Tackling and ideally preventing dementia requires a global perspective. In this episode, Matt & Donovan speak with Dr. Lindsay Kobayashi, a faculty member in the Department of Epidemiology at the University of Michigan School of Public Health whose research focuses on the social epidemiology of aging from a global perspective. Dr. Kobayashi introduces us to a whole new world of data available to help researchers tackle dementia as a global challenge.
More resources
- Lindsay Kobayashi Faculty Profile: https://sph.umich.edu/faculty-profiles/kobayashi-lindsay.html
- Article referenced in this episode:
- Kobayashi LC, Gross AL, Gibbons LE, Tommet D, Sanders RE, Choi SE, Mukherjee S, Glymour M, Manly JJ, Berkman LF, Crane PK, Mungas DM, Jones RN. You Say Tomato, I Say Radish: Can Brief Cognitive Assessments in the U.S. Health Retirement Study Be Harmonized With Its International Partner Studies? J Gerontol B Psychol Sci Soc Sci. 2021 Oct 30;76(9):1767-1776. doi: 10.1093/geronb/gbaa205. PMID: 33249448; PMCID: PMC8557836.
- CAPRA Website: http://capra.med.umich.edu/
Transcript
Donovan Maust:
Let's start with a few numbers. According to an estimate published in 2015, the global prevalence of dementia was projected to nearly triple between 2015 and 2050, growing from 46 million to over 130 million people globally. And of that worldwide share, 70% of those with dementia will be in low and middle income countries. Tackling and ideally preventing dementia requires a global perspective. Today, we are going to introduce you to a whole new world of data out there to help our listeners tackle dementia as a global challenge.
This new world is the International Partner Studies or IPS of our old friend, the Health and Retirement Study. I think Australia and Antarctica need to get it together, but otherwise, there are partner studies on every continent, which is pretty amazing. While you could do research with each country study as a standalone, some of the real potential comes from transnational comparisons. But for those to be the most useful, you want to make sure you're measuring the same thing.
For example, if you do delayed recall of 10 words, are the words that are used in the US equally difficult compared to the words on South Africa's list? To standardize assessments across the similar but not identical IPS studies, you need what's called data harmonization. Today, we're going to go global and talk about using harmonization to harness international data and inform our understanding of dementia.
Matt Davis:
I'm Matt Davis.
Donovan Maust:
I'm Donovan Maust.
Matt Davis:
You're listening to Minding Memory.
Donovan Maust:
Today, we're joined by Dr. Lindsay Kobayashi, the John G. Searle Assistant Professor of Epidemiology in the University of Michigan School of Public Health. Dr. Kobayashi investigates social and economic life course influences on cognitive aging, primarily using data from internationally harmonized longitudinal studies of aging.
In recognition of her contributions to the social epidemiology of cognitive aging in rural South Africa, she's an honorary senior researcher at a university in South Africa, which I think is one of the coolest affiliations we've had for one of our guests. Lindsay, welcome to the podcast.
Lindsay Kobayashi:
Thank you, Donovan. It's great to be here.
Donovan Maust:
Dr. Kobayashi was the lead author of the amazingly titled study, You Say Tomato, I Say Radish: Can Brief Cognitive Assessments in the U.S. Health Retirement Study Be Harmonized With Its International Partner Studies? That was published in the Journals of Gerontology Psychological Sciences. The citation for the article is linked to this episode, so please check it out. To get started, at a very high level for our listeners and frankly Matt and I who are not pros at this, what exactly is data harmonization?
Lindsay Kobayashi:
I think that's a great first question to start with, because it's really broad. Broadly speaking, data harmonization refers to all efforts to combine data from different sources and provide users with a comparable view of data from different studies. That is a definition given by the data sharing for demographic research project here at the University of Michigan. I like this definition because it's helpful when thinking about the broad mission of data harmonization, but like I said, there are also many discipline specific and subject specific definitions.
Now, harmonization may be as simple as cross-walking a variable across two different data sets. Coming back to thinking about the US Health and Retirement Study and its international partner studies, a great example is education. Education is a really common demographic variable that is measured in all of these studies, but the way in which it is asked about in study interviews and then recorded in data sets might be slightly different. For example, one study might record education as years of education attained.
Another study might record this variable as the highest degree level obtained. Of course, in different countries there might be different types of degree levels with different educational systems. There are resources such as the International Standard Classification of Education that provide crosswalks across different countries around the world with different education systems so that you can then harmonize disparate education variables across different education systems to come up with a comparable variable.
I think that's a really great basic and relatable example of data harmonization. But now when we are thinking about cognitive function, harmonization is a little bit more complicated. When I think about when my team and collaborators think about the harmonization of cognitive function data, we think about this essentially as a two-step process. The first step being statistical harmonization and the second step being statistical harmonization.
Pre-statistical harmonization, which is what we did in this Tomato Radish paper, which is what we've been referring to it internally whenever we talk about it, we refer back to this paper a lot in our ongoing harmonization work. Pre-statistical harmonization involves determining which cognitive test items are common and which are unique across countries or studies. And that is a really actually long and much more complicated process than it might seem at face value.
Statistical harmonization involves deriving harmonized factor scores using the methods that we use, which I know we'll talk about later, for either general cognitive function or specific cognitive domains. A harmonized score means that it is on the same scale and represents the same underlying construct of generalized cognitive function or whichever specific cognitive domain you want to be measuring across all of the different countries or studies that are being included.
Donovan Maust:
To go back to the... I like simple examples, and so if we go back to the years of education example that you gave, how do you know if you've harmonized it right? How do you know if you've come up with the right answer when you're combining these or comparing them?
Lindsay Kobayashi:
That is a really good question. Honestly, I'm not sure that anyone really knows that answer. There's actually a lot of different assumptions that we make when doing harmonization regardless of what the thing is that we are harmonizing. There's often no gold standard when we're doing harmonization. I think with education, what we have done on our team is, like I mentioned earlier, we have used the International Standard Classification of Education to crosswalk the data that we get across different countries, but we often still are making assumptions even there.
For example, the English Longitudinal Study of Ageing captures education in a really complicated way, or at least I think it's complicated because I didn't grow up in the British education system, which is really different at the public school level compared to the United States or even Canada, which is where I'm from. Their education system has changed over time. Sometimes when people are missing data on what degree they've attained, those information such as what age were you when you left school.
We want to use that information. We don't want to have missing data for people. We make an assumption about, okay, if you left school at age 16, then you probably finished with this degree level or with this grade. Even when something seems straightforward, there's often assumptions that we have to make. In our team, we do a lot of buddy checks. We meet weekly as a team.
I'll say that in addition to harmonizing all of this cognitive function data from the International Partner Studies, which is what we spend the bulk of our time on, we're actually also harmonizing data on what we call X variables, all of the risk factor data, all of the covariate data we want to be putting into our models. Those also have to be harmonized and you want to do a good quality job. I think about harmonization as garbage in, garbage out, which is probably a phrase you've heard when thinking about pooling data or running meta-analyses.
The same principle applies here. A lot of team meetings, a lot of buddy checks, a lot of independent coding. We're really explicit about the assumptions that we make and transparent, and we hope we do the best job possible with
Matt Davis:
With harmonization, I'm assuming that usually the motivation is to enable you to do new comparisons, but sometimes do people just harmonize data just because they want to increase their sample size, or is it more about comparisons?
Lindsay Kobayashi:
Yes, that is a really great question, and I think those are our two related, but different purposes for harmonized data. One really is meta-analysis or pooled data or pooled analysis where you really want to increase your sample size. I think that's something to be really careful with when thinking about doing so in the context of pooling data on aging, especially thinking about cognitive function, impairment, dementia as your outcomes across different countries.
Pooling data across populations makes sense if you really think that the effect you are trying to estimate is the same across all the different countries. There's no heterogeneity in the effect across the different populations that you are pooling data from. The opposite would be there is heterogeneity in the effect that you're estimating across countries, and that would be effect modification by country or by population. A great example would be the question, what is the effect of education on cognitive function?
Do we think it's really the same across the many different countries for which we have International Partner Study data on? The point estimate is the exact same with our harmonized education variable and our cognitive function variable, even though we know that education systems are really different across different countries, the curriculum are really different, the utility of education, what happens to you after you leave the education system that might affect your cognitive aging, what are job opportunities like.
All of those things might modify the effect of education on cognition across countries. If you think there is that kind of effect modification, you might not want to do a pooled analysis where you pool all your data into a single model and spit out one effect estimate. You might want to do a comparative analysis, like you said, and compare the effect of education on cognition across different countries with different education systems.
But if you really think there is one true effect estimate and it's operating in the same way across all the different populations and settings that you're studying, then yes, great, pool your data. A kind of analogous concept is meta-analysis. You can have a random effects meta-analysis where you assume there's some heterogeneity in the association across the different studies that you are pooling together in your meta-analysis or a fixed effects meta-analysis where you really think there's truly one effect, and all these studies are triangulating at that same effect.
Matt Davis:
Do people ever adjust for different factors when they're creating a harmonized measure, or is that kind of a no-no?
Lindsay Kobayashi:
What do you mean?
Matt Davis:
If you're trying to, I don't know, create a measure across different populations, did you ever control for other population factors and things built into the measure that you're harmonizing?
Lindsay Kobayashi:
Oh, I see what you mean. I guess I would think about that as norming the factor for age, sex, whatever. With cognitive function, no, we don't do that. We simply use the individual cognitive test items that have been included in the study interview for each population. Well, I suppose there are... The complex details of the analysis I'll give. The analysis to give a harmonized cognitive function score we'll get into, but essentially we would just use the information on the cognitive test item.
I think when you adjust a measure for another factor like age or sex, et cetera, you remove the ability to look at that factor directly in relation to the measure that you've just harmonized. If you adjust for age, then you've equalized cognitive function out across age groups, and you can't look at the effect of age on cognitive function, whether that's in a single population or cross nationally. When harmonizing cognitive function data, we actually want to look directly at the associations of our harmonized factor score with things like age, sex, education as an issue of criteria and validity.
Because we know that cognitive function tracks so closely with age, are we seeing the expected associations, for example.
Matt Davis:
Many of our listeners are already familiar with the Health and Retirement Study, but they're likely not as familiar with some of the International Partner Studies out there. I was wondering if you can tell us a little about some of those other studies that you looked at.
Lindsay Kobayashi:
Sure. The US Health and Retirement Study has a network of International Partner Studies around the world. And like Donovan said in the introduction, I think it touches every single continent, except for Antarctica, as well as Australia and New Zealand. In the Tomato Radish paper, we included data from the US Health and Retirement Study, as well as 11 of its International Partner Studies across 26 countries.
Some of the International Partner Studies include multiple countries such as the share study in Europe and the SAGE study, which includes several different countries around the world. SAGE stands for the Study on Global AGEing and Adult Health. All of these studies have really fun acronyms such as in China, we have CHARLS, the China Health and Retirement Study. In England, we have ELSA, the English Longitudinal Study of Ageing. My personal favorite is in India, we have LASI, which is the Longitudinal Ageing Study in India.
All of these studies, what makes them International Partner Studies is the fact that they have study designs and measures that are as harmonized as possible. These are longitudinal studies of aging. The baseline age will vary by country. Some of them go as young as I think 45. I'm actually not sure what the youngest age is, but I think that's just to account for those different life expectancies across different countries. We might consider mid-age starting at an earlier period in life in countries with shorter life expectancies, for example.
We want to capture some of those changes. Many of the measures, like I said, are harmonized. The ultimate goal of this network of International Partner Studies is to have data that are comparable so that we can do substantive comparative analysis to get a really comprehensive picture of what the social, economic, behavioral, and health related implications and resiliencies and opportunities are associated with aging around the world.
Donovan Maust:
Across these studies, there's an enormous amount of data collected by each of them. Why focus on cognition?
Lindsay Kobayashi:
As I'm sure many listeners of this podcast are aware of and I think what you did a great job introducing in the beginning, Donovan, the prevalence of dementia is rapidly rising worldwide. This is due to population aging. Because of this, understanding cognitive health is really important in the HRS and I would say always has been since the 1990s in the HRS.
This is also true in the International Partner Studies given that by 2050, nearly three-quarters of all dementia cases around the world are projected to occur in low and middle income countries which have very large populations such as India and China, and they are rapidly aging. Cognitive health is really of key importance given this really rapidly rising global burden of dementia, which is currently having a changing distribution around the world.
Donovan Maust:
There are a lot of different cognitive measures in the HRS, from self-reported questions to more valid assessments. Which ones did you use?
Lindsay Kobayashi:
We used the neuropsychological measures, which I think you're referring to as the more valid assessments that are objectively... They represent objectively measured cognitive function as opposed to a self-report, such as how would you rate your memory on a scale of one to five? We used all of these neuropsychological cognitive test items that were available in the HRS main interview assessment, and we did the same with all of the International Partner Studies.
If you go online and look at the paper, there's a great supplemental table that visualizes the coverage of cognitive test items across all of the International Partner Studies that we included in our analysis. I'll say briefly, we identified 53 unique cognitive test items, and the most common of which across the studies was the ability to state the current day of the week. This is an important item to assess orientation to time and immediate recall of a list of 10 words read out loud by the interviewer, which is a great item that assesses episodic memory.
We found that all of the studies had at least four items in common with at least one other studies, but most of the International Partner Studies had several items that were completely unique and not in common with any other studies. I'll also say that these measures were from the main interviews of the US Health and Retirement Study and its International Partner Studies.
Listeners to this podcast may also be familiar with the Harmonized Cognitive Assessment Protocol, which is a newer innovation that has been implemented by the HRS and some, but not all of its International Partner Studies, which is a longer more in-depth cognitive assessment.
And like its title, it's really intended to be harmonized because the study developers did recognize that the cognitive assessments in their main interviews were, A, brief, and B, really inconsistent across all of the studies and they wanted to have a more common harmonized suite of measures that would more readily facilitate cross national comparisons.
I will also note that the HCAPs are not done on the full study samples like what we were able to include here, but on selected sub samples of each international partner study just because of the length and nature of the interview, which is quite involved for the HCAP.
Donovan Maust:
In the introduction for your paper, you briefly touch on the distinction between fluid and crystallized cognitive function. Could you just explain that distinction a little bit and the assessment that you're looking at, which types of intelligence or function is it focusing on?
Lindsay Kobayashi:
Yes, of course. Those phrases, crystallized versus fluid cognitive function, I think are used more commonly in the psychology literature as opposed to epidemiology, which is my field. I would also like to veer away from the phrase intelligence because there's a really long and controversial history of intelligence testing, not just in the cognitive aging dementia world, but I think that that is a concept that has a lot of scientific difficulties and has been used to justify hierarching people into different groups based on their perceived intelligence.
I think that has been very problematic in a lot of ways over time. What we are focusing on here is specifically cognitive function during aging and really thinking specifically about cognitive impairment and dementia. Most cognitive assessments that we use to classify cognitive health status capture fluid cognitive functions, and these fluid cognitive functions are those that are more sensitive to aging related change or pathology over time. These would be things like episodic memory, executive function, orientation, and things like that.
Crystallized cognitive functions would be those that are actually relatively stable with aging, so thinking about long-term stored memories or forms of knowledge.
Matt Davis:
From my understanding, some of the continuous measures for cognitive assessment just in the United States, there's issues when you go to collapse that to identify different functional status. I guess when you're harmonizing data, just big picture, are you trying to standardize the continuous measures? Because I can imagine as you think about that across countries and if you have those kinds of issues with how you identify people with a cognitive impairment, it just must get super complicated and difficult.
Lindsay Kobayashi:
Yes, we can talk about that. Here we are focusing on the continuous measure of cognitive function. This is distinguishing across the full range of functional ability. At the lower end of the distribution, we'll capture people with dementia and cognitive impairment. At the upper end of the distribution is people with normal cognitive function. And that is completely different from trying to classify impairment or dementia according to DSM thresholds or other types of thresholds for identifying status.
That is something we're working on right now. I'm also a part of the Gateway to Global Aging. For the Gateway to Global Aging, we do have an interest in coming up with harm... We should talk... I don't know, maybe we should cut this out. The Gateway to Global Aging Data is a whole different beast, but I would say we are working on harmonizing algorithms for cognitive impairment and dementia across all of these studies using the HCAP data.
This continuous HCAP score is part of that, but we also use data on functional impairments, informant reports, and in some cases validation using clinical dementia ratings from clinicians who review data. All I can say right now is it's extremely challenging to come up with a harmonized algorithm for cognitive impairment and dementia, even just thinking about the relevance of ADLs and IDLs, different living contexts, thinking about living in the United States versus England versus India versus Nepal versus China.
There's different levels of functional ability that are required to live independently in each place depending on what types of services and family supports are available. Another question there is, how relativist or absolutist do you want to be? And that's actually a bigger issue. That's an issue in harmonization regardless, but an especially big issue in thinking about cognitive impairment and dementia. I can suggest a guest to come talk to you about that if you're interested in those diagnostic classifications. It's a challenge.
Matt Davis:
You mentioned at the beginning of the episode that there's a lot of different approaches that people use out there when they try to harmonize data. Broad terms, what did you do? What approach did you take?
Lindsay Kobayashi:
The method that we used is confirmatory factor analysis or CFA within an item response theory or IRT framework. For listeners who are not familiar with confirmatory factor analysis, if you know linear regression, you can do confirmatory factor analysis. I'm going to refer to it as CFA. What we do in CFA essentially is we assume that we have several different indicators in our data that are measured. They are real. In this case, they are scores on each of the individual cognitive test items that are administered in the study interview.
Now, we think that those individual cognitive test items, which here I will refer to as indicators, are reflecting some underlying construct that is unmeasurable, which in this case is cognitive function. In the HRS study interview, the interviewer might ask the respondent, okay, what day is it today? What day of the week is it? What month is it? What year is it? Who is the current president? Okay, great. Each of those is an individual test item. They'll then read out a list of 10 words and ask the respondent to repeat them back immediately.
And then 10 minutes later in the interview they might say, "Oh, hey, remember that list of 10 words? Can you repeat it back to me again?" That's the immediate and delayed word recall, which assesses episodic memory. Those are two additional cognitive test indicators.
And on their own, each of those measures of orientation and those measures of immediate and delayed recall don't comprehensively tell you what a person's overall level of cognitive function is, but we administer these multiple different tests because we think each one of them is getting at a different aspect of a person's level of cognitive function. This underlying construct of cognitive function isn't measurable in and of itself, but we have these multiple indicators that are giving us an idea of a person's level of cognitive function when we put it all together.
And that is what confirmatory factor analysis is attempting to do. In the confirmatory factor analysis model, we would take a person's score on each of these measures. Some are going to be continuous, some are going to be binary, like what day of the week is it today? Did you get it correct or incorrect? That's going to be a binary item. The CFA model can handle both continuous responses and categorical or ordinal responses.
What we're going to do is we're going to take the common co-variation across all of these cognitive test items and use that common co-variation to construct what we refer to technically as a latent trait or latent variable. In this case, we're going to call it cognitive function. Each individual cognitive test item is going to have what's called a factor loading, which represents the strength of association between that individual cognitive test item and this underlying latent variable representing cognitive function that we have now just constructed.
A factor loading is analogous to a regular regression coefficient. A stronger factor loading is going to indicate that that particular cognitive test item co-varies more strongly with the other test items than another individual cognitive test item that might have a weaker factor loading. Individual cognitive test items that have a stronger factor loading mean they are more influential in determining the latent variable representing cognitive function in this case. Latent variables don't have a natural scale.
They're standardized. You can standardize them to whatever you want really. But in this case, we use a mean of zero and a standard deviation of one. It's this variable that is constructed from the individual cognitive test items to represent this bigger construct of cognitive function, which theoretically is what we think we are measuring. We feel pretty comfortable taking these cognitive test items and using their co-variation to generate the latent cognitive score. That's the basic machinery of what we do in harmonization.
It gets a lot more complex in a lot of ways that are probably too in depth to get into in this podcast, and that's where the Tomato Radish paper stops. We estimate all of the CFAs and we show the model fit statistics, but the next step would actually be to do what we call statistical harmonization, where, again, we are going to be estimating multiple CFAs, so one CFA model for each country, but we're actually going to allow each country to have different cognitive test items loading onto that construct of cognitive function.
That's our latent variable. As long as we have at least one anchor item, and that is a cognitive test item that we are theoretically confident performs in the same way across all of the different countries under study, so equal difficulty, equal discrimination of different levels of cognitive ability, we are going to use that anchor to help us to co-calibrate the cognitive test scores across all of the different countries that we are harmonizing, even if some of the individual cognitive test items vary across countries.
This works because we make that assumption that these cognitive test batteries are reflecting the same underlying latent construct of cognitive function. We can do this on a broad level like we did here in this paper for general cognitive function, and we can also do it on a domain specific level and split items or cognitive test items representing, for example, memory executive function or language.
Matt Davis:
But long story short, at the end of the day, everybody gets a measure, right?
Lindsay Kobayashi:
Yes. At the end of the day, everybody gets a score and that score has, again, no natural scale, has a mean of zero and a standard deviation of one. It's normally distributed.
Matt Davis:
I mean, there's no way to know whether it's valid or not, but by comparing the coefficients across countries, you can start to get a feeling whether it's behaving correctly.
Lindsay Kobayashi:
That's right. We use model fit statistics for CFA models in assessing... I don't know if validity is the right word. They're assessing the model fit.
Matt Davis:
Reliability maybe.
Lindsay Kobayashi:
Yeah. When we think about reliability, that's a little bit more about precision across the scale or across, sorry, the range of the latent cognitive variable. But the model fit statistics for the confirmatory factor analysis essentially are telling us how well all of those cognitive test items are hanging together.
Donovan Maust:
Are there approaches other than confirmatory factor analysis to do harmonization, or is that the tool when you're harmonizing?
Lindsay Kobayashi:
There are other approaches. When thinking about cognitive function, something a lot of people do is just take a simple summary score and add it up, and then zed standardize it to get it on a common scale. That's easy. That's not a harmonized score for many reasons. Another approach with cognition data could be equipercentile equating, and that's not an approach that we necessarily need to do here.
We feel that when it comes to the International Partner Study data, both the main interview batteries as well as their HCAPs, the approach that we're using with CFA for statistical harmonization is the most flexible in allowing both continuous and non-continuous variables in terms of the individual cognitive test items and also for testing what we call differential item functioning, which is a type of analysis that we're working on now, which is a step beyond the Tomato Radish paper.
But overall, we think confirmatory factor analysis is the most flexible and we can do the most analytically in terms of testing, like you said, the reliability of our models and testing for differential item functioning.
Donovan Maust:
How successful were your analysis, would you say?
Lindsay Kobayashi:
In this paper, what we found was that six out of the 12 International Partner Studies had models that were of acceptable fit according to the standard CFA model fit statistics when coming up with a unidimensional factor analysis model where we are estimating one single latent variable representing cognitive function. That's actually not great. I'm not sure if I would say that is a success, but I'm not sure if that's anything to do with the methods. It's just the data.
However, when we estimated by factor models, so here now we're going to be estimating more than one latent variable simultaneously from our cognitive test items, that's when we actually found that all 12 out of 12 studies had acceptable model fit according to standard model fit statistics. With the bifactor models, what we did was in addition to estimating a latent variable for general cognitive function, we also estimated a latent variable representing memory. And that is because standard cognitive test items assessing memory have really high correlations with each other.
Immediate recall of 10 words correlates really strongly with delayed recall of 10 words, so much that the correlation between those two items almost subsumes a latent variable for general cognitive function. But when you pull them out into their own factor and allow them to hang together separately from the other items, then we get a better model fit. In that sense, I would say our analysis was successful. If you read the paper, we concluded that yes, the data in these cognitive batteries in the main interviews of the International Partner Studies can be harmonized.
We should be pulling out specific bifactors for memory. But the other thing is that there is a lot of non-overlap of cognitive test items and we cannot harmonize scores for specific cognitive domains such as memory or executive function because we have too few items per domain. We had to keep it to general cognitive function. In that paper, we concluded that the harmonized cognitive assessment protocol probably provides more opportunities for harmonization given it's longer battery that allows us to look at domain specific cognitive function.
Donovan Maust:
Do you think there would be value in doing harmonization of the cognitive assessments using alternative approaches, or do you think this has established what needs to be established in terms of harmonization of cognitive assessment across these partner studies?
Lindsay Kobayashi:
I'm a big fan of sensitivity analysis and to comparing results when using alternative methodologies. I do think there would be value in doing that. Even something as simple as how does a simple summary score compare to or how do results differ when using a simple summary score for cognition as opposed to the harmonized factor scores? What happens if we use equipercentile equating versus this IRT based approach? I think that there's opportunity for good methodological papers to do that.
Donovan Maust:
I guess, how do you think about the implications or the application of your findings? Is it that the studies should be using different types of assessments, or how should our listeners think about what to do with the work that you've done?
Lindsay Kobayashi:
Here's what I think. I think that this harmonization work as applied to studying cognitive function during aging is really important because the cognitive assessment in the Health and Retirement Study, both in its main battery as well as much of the content of its harmonized cognitive assessment protocol, which I know many data users are using now and have interest in using now, have largely been developed for Western English speaking and literate populations.
Those cognitive test items in the interest of having harmonized measures across this International Partner Study network have been adapted to populations around the world that have had lower opportunities for education, have high prevalence of illiteracy, and where the cognitive test items have been translated into different languages, as well as adapted for cultural relevance. A great example there is there's a test item that asks the respondent to recognize a cactus.
Now, cacti are not indigenous to all regions in the world. In the LASI study in India, that item was adapted to be about a coconut. In contrast, in China, that item was not adapted at all. My understanding I've learned is that cacti are really also not indigenous in China. And now this item is actually really easy. Most people get this item right and are able to identify a cactus if they are not experiencing some kind of cognitive impairment or dementia.
However, we see that in China there's really high rates of getting this item incorrect, much higher than we would expect the prevalence of cognitive impairment or dementia to be. Whereas in India, with the item that's been adapted to a coconut, people are performing really, really well. As an interesting side note, we also see some regional variation across Northern to Southern India in terms of rates of getting this correct. That's one example of a cultural adaptation.
I think without really extensive thought in terms of which cognitive test items really are performing well and not well across different populations, given what we know about how they've been adapted, I think we do have to be really thoughtful and use the data fairly. And that is the ultimate reason why we are doing this statistical harmonization.
I didn't talk very much about the pre-statistical harmonization process, but this involved working with cultural neuropsychologists, as well as the primary field work teams from each of these studies around the world to get a really clear idea of how items were administered in the field, what were the visual prompts, if any, that were included, were respondents primed or prompted to respond, if they failed to respond immediately, things like that.
And that helped us to identify or make determinations about which items we thought really were comparable across countries and which ones weren't, even if it appeared like they were based on the study documentation and variables. This is implications for how we model the items.
Items that are comparable when we are harmonizing the data, we fix them to have identical factor loadings across countries because we think that particular cognitive test item should influence cognitive function, whether that is general cognitive function, memory, executive function, or something else similarly across settings.
But if we know that a particular translation or cultural adaptation or just logistical difference that happened in its administration changes or might change the way that someone interprets the question and response to it, then we want to allow the factor loadings to be different across countries because we think that is really a different item.
The statistical harmonization is really about achieving this balance between items that we assume to be comparable or that we think based on our best information are comparable, these are the anchor items that we fix versus items that are freely estimated, because we do need at least one anchor in order for our models to run. But anytime we designate an item to be an anchor, we have to make an assumption that might be quite strong because there can be differences in interpretation and administration baked into these items that we as researchers just can't see or don't know about.
I think that's apparel of using a simple summary score. Whereas with the statistically harmonized scores, we allow items to load equally or differently onto our final variable for cognitive function, that latent cognitive function score, depending on what we know about how they were adapted and administered. We are actually producing statistically harmonized factor scores for general and domain specific cognitive function for all the studies that have implemented HCAPs to date. That's one of my main research grant is focused on right now.
We hope that data users will use these scores. We're in the process of making them publicly available through primary study websites, as well as through the Gateway to Global Aging Data right now. I think that having that basic understanding of confirmatory factor analysis is helpful in terms of understanding what that statistically harmonized factor score represents. There are also other issues in terms of thinking about what is a good research question for a substantive cross-national comparison as well.
I think that's incredibly important when thinking about cognitive function as an outcome because the measurement of cognitive function can be so heavily influenced by things like language and culture and economic development that we want to be really careful. I would encourage data users to think really carefully about the implications of using harmonized data across different countries and the ways in which measures might be affected by adaptations, and then how to best account for those adaptations in their analysis.
Matt Davis:
What's next for your own research? I'm curious if you're going to continue to work on the methods behind harmonization or actually start using them for surveillance or something else?
Lindsay Kobayashi:
We're using them. We have our harmonized factor scores. We actually have a pre-print that's up right now. The paper is also under review at a journal. We hope to get those results out there for the full statistical harmonization of HCAP data. We're using the harmonized scores. I talked about education and cognitive function earlier on in the podcast. We're working across a team to compare the associations of education and cognition in men and women across countries at different levels of economic development.
We're looking at other socioeconomic factors in relation to cognition across countries such as main lifetime occupational skill level. We're also starting to dig into cardiovascular risk factors and how that relates to cognition across different countries, because we're actually seeing some inverse associations and they're inconsistent across countries at different levels of economic development, which is really interesting and a big puzzle for us to figure out. I'll say at this point that this is really a team science project.
It's hugely collaborative. It's a ton of work. I'm leading this work alongside Dr. Alden Gross at Johns Hopkins University, who is also an epidemiologist, as well as several other investigators from different universities in the country. In addition to the substantive analysis, we're also continuing to expand our pool of data with respect to the HCAP. The HCAP is continually being implemented in more and more countries around the world. And as those data become available, we are going to be adding them to our harmonization item bank.
Matt Davis:
We're also curious too if there's a story behind the title of your article.
Lindsay Kobayashi:
That's a great question. I do not take full accountability for that title. The title actually came from one of our co-authors, Maria Glymour. I remember this so well because we as an authorship team had been brainstorming what should the title of this paper be. I remember we had this long email thread going back and forth, and I remember I was standing in the checkout line at Costco absentmindedly scrolling through my phone, and this email popped up from Maria that said, "What about You Say Tomato, I Say Radish?"
And I knew that was the perfect title, and I don't know exactly what her thinking was when she came up with it. I feel it was immediately intuitive to all of us on the team though. My interpretation of that title is that, well, first of all, it's obviously a play on words, right? You say tomato, I say tomato is a common saying, and that's kind of like a knowable cultural difference in language or pronunciation of the word tomato or tomato, if you will. However, when you see or read, You Say Tomato, I Say Radish, that catches the reader off guard.
That's not what you expect from that play on words. I think that speaks to the kind of unknowable unknown when it comes to harmonizing cognitive function data across countries. There are certain things that we know aren't going to perform as well in different contexts, such as naming a cactus. That's not going to perform as well in regions where cacti are not indigenous and people don't see them or think about them all the time.
But there's probably also some unknowable unknowns that we as researchers, and coming from the perspective of being highly educated English-speaking researchers and specifically here in the United States, just aren't going to know in terms of how people in different countries and contexts are interpreting cognitive test items. I think that phrase, You Say Tomato, I Say Radish, really speaks to that challenge of harmonization as researchers.
Donovan Maust:
Anything we haven't touched on that you think would be good before we go?
Lindsay Kobayashi:
I think we've covered it. I think that I could talk about harmonization of cognition data for a really long time. I think that as researchers who are interested in understanding risk and resilience for dementia, one of the last things that we would want to do is have our work bleed into that controversial history of intelligence testing, which attributes differences in cognition to innate or inherent differences between people or between groups.
I think that the dementia research community is so exciting and so diverse and has such a positive focus to understanding the causes of dementia and thinking about trying to delay or prevent its onset. We're really shifting also to thinking about resilience of the brain to aging and thinking about modifiable risk factors and thinking about what factors produce unjust inequalities in brain health in later life.
I think that the International Partner Studies of the Health and Retirement Study give us a hugely powerful tool to take a global perspective on risk and resilience factors for dementia. Having data from a diversity of global regions might help us to identify risk and resilience factors that we cannot identify from a single population alone, for example. They can also allow us to ask how known risk and resilience factors might play out differently in different social and economic settings.
I think there's huge opportunity in that. But I also think at the same time, we have to be really careful in understanding how cognitive data are measured across different populations, how test items have been adapted, and how to think about the implications for the data that we use.
Ideally, I do hope that users will use the harmonized factor scores because we've tried to really be thoughtful in terms of accounting for different types of adaptations, whether that is a cultural adaptation, a linguistic adaptation, a logistical adaptation in terms of estimating our factor scores, and then at the end to be really thoughtful in terms of interpreting the data and attributing differences in cognitive function to some of the bigger structural factors that might shape population level differences in cognitive health.
Matt Davis:
Thank you so much for joining us, Lindsay. I feel like I just have learned a tremendous amount and you're doing a tremendous amount of work. That's amazing. We appreciate you taking the time to join us. Thanks to everybody who listened in.
Lindsay Kobayashi:
Thanks for having me.
Matt Davis:
If you enjoyed our discussion today, please consider subscribing to our podcast. Other episodes can be found on Apple Podcasts, Spotify, and SoundCloud, as well as directly from us at capra.med.umesh.edu, where a full transcript of this episode is also available. On our website, you'll also find links to our seminar series and data products we've created for dementia research. Music and engineering for this podcast was provided by Dan Langa. More information available at www.danlanga.com.
Minding Memory is part of the Michigan Medicine Podcast Network. Find more shows at uofmhealth.org/podcasts. Support for this podcast comes from the National Institute on Aging at the National Institutes of Health, as well as the Institute for Healthcare Policy and Innovation at the University of Michigan. The views expressed in this podcast do not necessarily represent the views of the NIH or the University of Michigan. Thanks for joining us, and we'll be back soon.

Listen to more Minding Memory podcasts - a part of the Michigan Medicine Podcast Network.


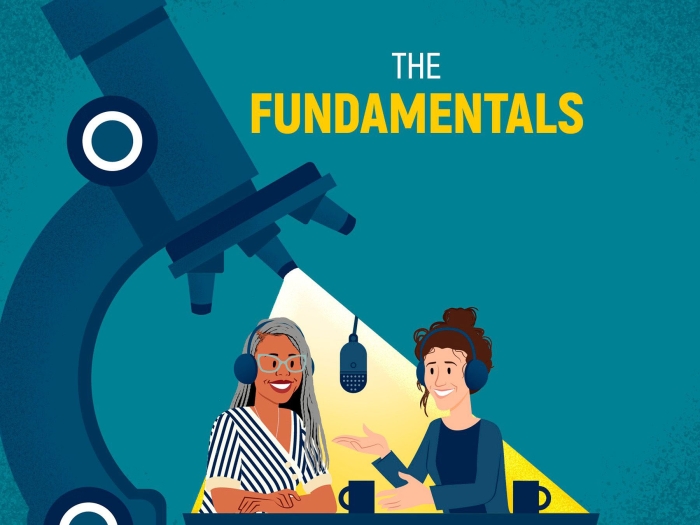
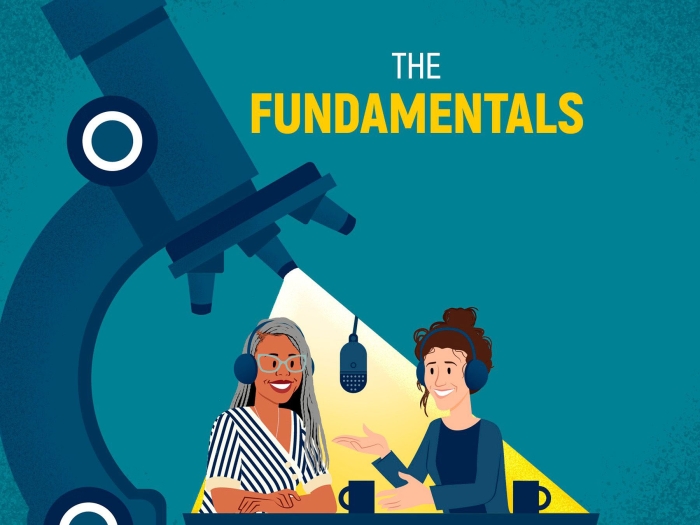
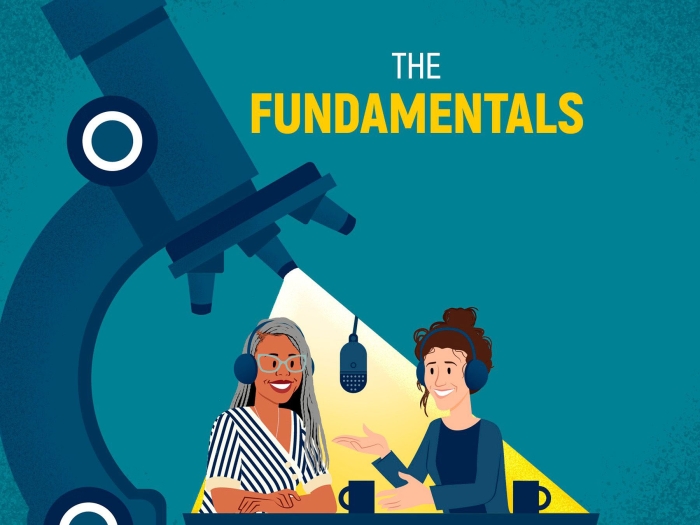
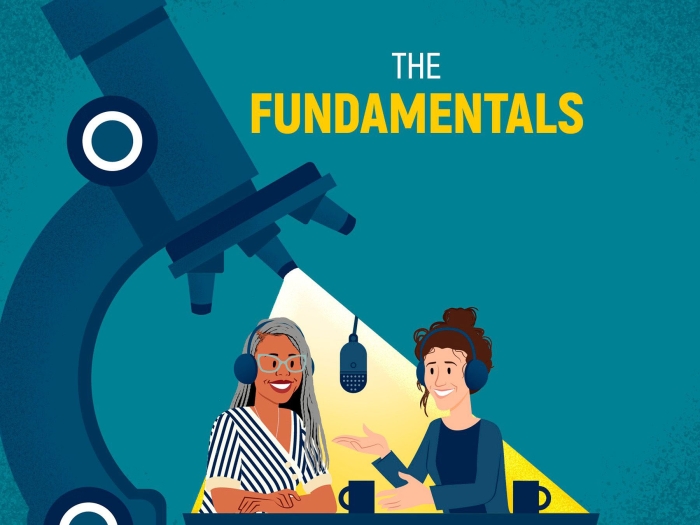